Are you what you Tweet? OPF releases Twitter experiment results
The Online Privacy Foundation (OPF) encourages people to get online and consider all the great things social networking sites could do for them. But the evidence is growing that we need to think harder about how we best share personal information online and question how that information is used.
The OPF has released the results from our study into how well anti-social personality traits can be determined from how individuals use Twitter. The results show that there are a number of statistically significant relationships between personal Twitter activity and certain personality traits, such as narcissism, Machiavellianism and psychopathy. But these relationships are relatively weak, especially when applied to individuals. The results do indicate that wider social trends may be more readily determined. This has important implications for how Twitter and other social media services are used to understand individuals and society as a whole.
The full results of the study are available in this paper, which was the result of an OPF collabortation with Rachel Boochever (Cornell University) and Gregory Park (University of Pennsylvania).
The main conclusions
Our results add to a small but growing body of research that suggests what people do on social media does appear to provide some clues to their underlying personalities. Currently these clues are far from perfect and in our opinion should not be used for trying to determine the personality of an individual – the chance of getting it wrong is just too high.
However, as behavioural research with social media matures, it will likely offer important insights into the levels and variability of anti-social behaviours within and between social groups. This field of research may be of great benefit in examining changes in society over time; using social media to monitor shifts in anti-social (and social) behaviour may prove to be a possibility.
Our results also indicate that while users may be careful about the content they post on Twitter, the words they use may reveal more about their personalities than they would wish. This raises critical questions around the possible need for regulatory controls and/or raising awareness among users to prevent the misuse of information derived from Twitter and other online social network activity.
As an organisation, we are concerned that everyday people will be judged or pigeonholed by what they do and say online in undesired ways, with a large number of those judgements being wholly inaccurate. Many people use the argument that “I’ve done nothing wrong, therefore I have nothing to hide”; yet our research demonstrates the number of mistakes that can be made through social media vetting, and that people risk being incorrectly penalised for their online behaviour.
How we did it
2,927 Twitter users from 89 countries took part in our study, providing self-reported ratings on the Short Dark Triad (SD3) questionnaire providing measures of narcissism, Machiavellianism and psychopathy; and the Ten Item Personality Inventory (TIPI), providing measures of openness, conscientiousness, extraversion, agreeableness and emotional stability.
For each individual, we extracted a total of 586 features from their Twitter profile and usage, including friends, followers, number of Tweets and the frequency of use of pre-defined words.
These features were used to examine whether there were statistical relationships between Twitter usage and personality.
Finally, we examined how well computer models would succeed in trying to predict how someone scored in terms of their personality traits. We hosted a data science competition on Kaggle.com, where data scientists from around the world competed to produce the best predictive models. The results of the Kaggle competition are reflected in this article and paper.
We also partnered with data scientists at Florida Atlantic University who developed new machine learning techniques. The techniques developed by Florida Atlantic University are included in the slides, but will be included in a separate paper.
Both papers have been accepted for inclusion in the 11th International Conference on Machine Learning and Applications in December.
Why we did it
There appears to be a number of increasing risks that our online social network activity will either be misinterpreted or simply used against individuals in unintended ways. We also recognise there are a number of opportunities to use online personal information to better understand society. This experiment and our 2011 Facebook study were intended to help us discover the issues we need to discuss as a society, so we are better equipped to make the right decisions about them.
A striking example is the growing trend of hiring managers vetting potential employers based on their social media activity. If it really is possible to determine personality through social media, then people need to know. But if it’s not readily possible then it raises an important question regarding the practice of social media vetting. The potential ramifications of social media vetting are vividly portrayed in this recent guardian article.
So why did we choose to examine anti-social personality traits, and psychopathy in particular, in the Twitter experiment? While we hope that others will use our research to look at wider issues, we decided to focus on the more anti-social personality traits of psychopathy, Machiavellianism and narcissism, often referred to as ‘The Dark Triad‘, in order to critically examine a number of topical and pressing questions in the media and academia around:
- Public understanding of psychopathy
- Whether it is possible to spot psychopaths and/or psychopathic traits from Twitter usage
- Perceptions that detecting personality from social media is infallible
Our discussion of recent press coverage on our initial findings provides more background (here).
What we found
Our study showed that there were some statistically significant correlations between Twitter usage and a user’s personality. But it was personality prediction that we were most interested in studying.
The images below explain how well our predictive models did at detecting people scoring in the top 10% of our psychopathy test. Fig 1, below shows what a 100% accurate prediction would look like. The people who scored in the top 10% of the SD3 test shaded red and everyone else shared blue.
Fig 2, shows that a random, uneducated guess (the toss of a coin) would correctly identify half of the high scorers, but would also catagorise half of the high scorers as low (and vice-versa for the low scorers).
Fig 3, below, shows how the prediction would look if we just guessed that everyone scored low. This is a reasonable assumption, and gives us an impressive looking accuracy of 90%. However, the model completely fails to identify any high scorers. This is an important point as accuracy is a common way to measure the performance of predictive models. For this reason, it is often appropriate to use multiple criteria to measure how well a predictive model really works.
Fig 4, shows the improvement gains from the winning Kaggle model. This is clearly better than both the random guess and the educated guess, but is still far from perfect.
Based on these results, we concluded that machine prediction of psychopathy is not yet accurate enough to be used to determine whether someone scores high in psychopathy or not.
However, the results are a reasonable improvement over a toss of a coin guess. Considering the case of a hiring manager wishing to avoid the kind of employees who scores high in psychopathy tests, they’d certainly be moving the odds in their favour by using models like these. Using our group of 100 potential employees, a random guess would see that the chance of hiring a high scorer is roughly 10% where this model would reduce that figure to roughly 5%. If the hiring manager was not concerned about rejecting roughly 30 low scorers, this strategy may be effective, especially if subsequent hiring steps can root out the remaining 5%.
Note: The techniques developed by Florida Atlantic University and to be shared at the 11th International Conference on Machine Learning and Applications slightly improve the ability to predict high scorers. Using our example above, the chance of hiring a high scorer would be reduced from just over 5% to just under 4%.
The largest predictor of psychopathy based on Twitter usage, was swearing/profanity in Tweets. The JobVite 2012 survey shows that profanity in Tweets generates a negative reaction for 61% of hiring managers (top was reference to illegal drugs at 78%). Our research suggests that if hiring managers want to reduce the risk of hiring people scoring high in psychopathy, the JobVite criteria may, quite by chance, be appropriate. But they’d incorrectly omit a high number of low scorers.
Results from our experiment for the three ‘Dark Triad’ and the ‘Big Five’ personality traits indicate that some traits may be easier to predict than others. See our paper for more details.
The implications for individuals are high as a digital past can be very difficult to erase. As mentioned in this recent article about privacy, do we really want to live in a world where “nothing is ignored, nothing is forgotten, nothing is forgiven”?
A less objectionable use?
Since the results are a reasonable improvement over a toss of a coin guess, it may be worth exploring whether models such as these could be used to examine societal trends over space and/or time; e.g. are we becoming more ‘anti-social’? Is one group more ‘anti-social’ than another? This may for instance have relevance to the growing scientific and political interest in the measurement of well-being and other areas of public policy. To demonstrate what this might look like, the following diagram (Fig 5) and accompanying table (Table 1) were derived from our results. It indicates where value in future research might lie. Together, they show the actual and predicted SD3 psychopathy scores for people with either UK or USA in their Twitter profiles. The median and upper values are consistently higher in both the UK predicted scores and actual scores when compared with the actual and predicted scores of participants from the USA. i.e. of the Tweeters who took part in our study, those in the UK scored slightly higher than those in the USA.
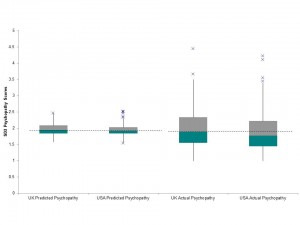
Fig 5. Box-Whisker Plot of Actual and Predicted SD3 Psychopathy Scores (UK v USA)
Click on image to see a larger version
Our Paper and Presentation Slides
You can find our paper here and you can find presentation slides, complete with speaker notes here (Note: presentation slides are 3MB and we may be changing them over time).
The slides were first presented at DEF CON, Las Vegas, NV on July 29th 2012 and again at 44Con, London, UK on September 6th 2012.
If you are interested in further information about the experiment, the work of the OPF, or a research collaboration with the OPF, please get in touch with us via our contact page.
Chris Sumner and Matthew Shearing, OPF Co-Founders